Abstract
This seminar focuses on fixed-location forecasting of physical parameters collected by sensors, with a novel methodology for dynamically integrating multiple sources of training data. The forecasts of fixed locations with a generalized model aid in cases where data is missing or noisy due to anomalous local conditions. Due to careful collection and modeling of data from various agencies, multiple sources of data exist for any given physical parameter. This suggests that methods which automatically make use of more than one source of data are intrinsically valuable. The talk covers key background concepts, dataset availability, machine learning surrogate modeling, and techniques for merging multiple data streams. To illustrate the approach, several detailed case studies will be presented.
Biography
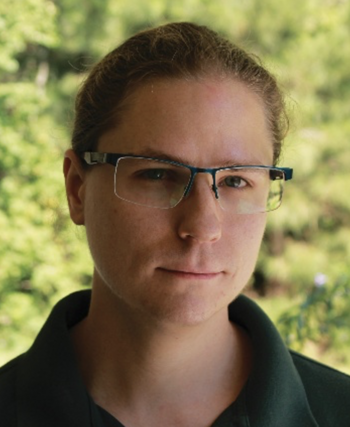
Austin Schmidt earned his M.S. in Computer Science in 2021 and is a recipient of the prestigious SMART scholarship from the Department of Defense. He is currently pursuing a Ph.D. in Engineering and Applied Sciences at the University of New Orleans. In addition to his doctoral studies, he conducts machine learning research at the Canizaro Livingston Gulf States Center for Environmental Informatics (GulfSCEI), focusing on developing models to forecast and simulate oceanographic and climatological parameters.