Abstract
Undetected defects in culverts and sewer pipes pose significant risks to public safety, leading to infrastructure collapses, flooding, and transportation disruptions. Traditional manual inspections are time-consuming, costly, and prone to human error, while existing automated methods struggle with occlusions, irregular defect shapes, class imbalances, and high computational demands. To address these challenges, this dissertation develops advanced semantic segmentation systems that automate defect detection, significantly improving efficiency and accuracy.
This research introduces a series of innovative models designed to overcome these challenges in underground infrastructure inspection. Using dual-attentive mechanisms, sparsely connected blocks, and depth-separable convolutions, these models improve segmentation performance and effectively handle data imbalances and variations in object characteristics. A key advancement is the Asymmetric Network, which optimizes the traditional encoder-decoder architecture by employing a more efficient decoder for upsampling and reconstruction. This approach reduces model complexity and computational costs while maintaining high accuracy. As a result, the proposed system accelerates both training and inference, reducing inspection time by up to 80% and generating significant cost savings, making it ideal for resource-constrained environments. This dissertation lays the groundwork for future research in adapting deep learning models to real-time inspection scenarios, integrating multi-modal data sources, and improving model generalization across various infrastructure types. At the same time, it delivers a practical, high-performing solution for automated infrastructure inspection, advancing defect detection and offering real-world value for municipalities, engineers, maintenance teams, and infrastructure management organizations.
Advisors:
- Dr. Md Meftahul Ferdaus
- Dr. Mahdi Abdelguerfi
Biography
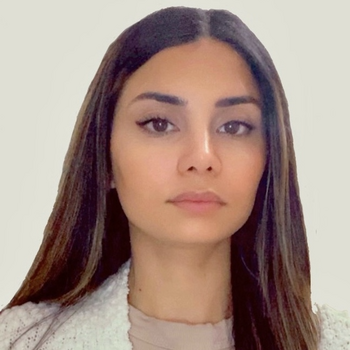
Rasha Alshawi was born in Baghdad, Iraq. She pursued a Ph.D. in Engineering & Applied Science at the University of New Orleans, focusing on deep learning, computer vision, and their applications to infrastructure systems. Rasha is a member of the Canizaro Livingston Gulf States Center for Environmental Informatics (GulfSCEI), where she conducts research focused on automating the inspection and monitoring of underground infrastructure. In collaboration with the U.S. Army Corps of Engineers, her work aims to enhance maintenance efficiency and safety by developing deep learning models for defect detection in critical infrastructure.