Abstract
Physics-informed neural networks (PINNs) can serve as fast, equation-aware surrogates for the computational models used in river, levee, and subsurface analysis. By embedding governing hydrodynamic and hydrogeologic equations within the learning process, these surrogates deliver accurate results in seconds while preserving physical fidelity. This seminar surveys recent advances spanning surface flow, seepage, and subsurface property inference, and shows how order-of-magnitude speed-ups unlock rapid analyses and risk-aware decision support for large river systems.
Biography
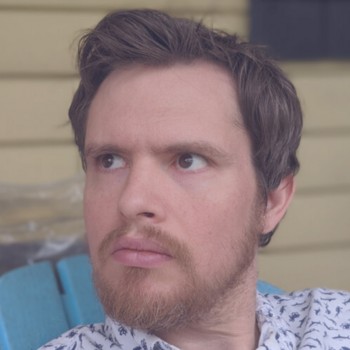
Ted Holmberg is a Postdoctoral Research Associate at the Canizaro-Livingston Gulf States Center for Environmental Informatics (GulfSCEI) and previously served as a research associate at the U.S. Naval Research Laboratory. He earned a B.A. in Philosophy, an M.S. in Computer Science, and a Ph.D. in Engineering & Applied Science from the University of New Orleans, where his 2024 dissertation introduced the ROBUST framework for optimizing spatiotemporal observer networks. Ted now develops physics-informed ML surrogates and visual analytics for hydroinformatics applications.